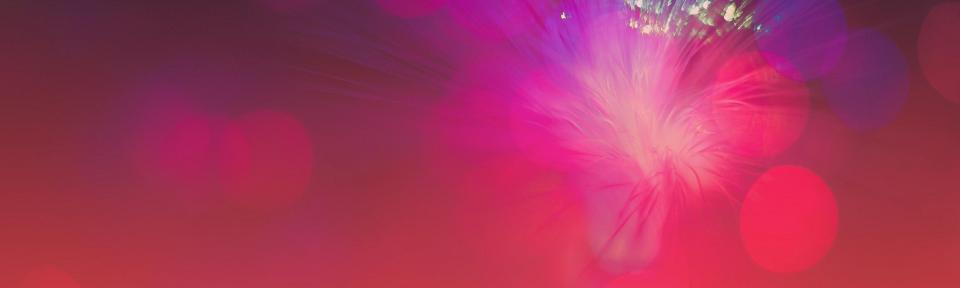
Machine learning and physical therapy don’t seem like they’d go hand in hand. But a recent PhD graduate from the Rehabilitation Science Program is using her data skills to help harness machine learning and give providers and clinicians more accurate patient predictions.
Danica Dummer, PT, DPT, NCS, PhD, works as a clinical consultant lead for Medical Group Analytics, a branch of University of Utah Medical Group. We caught up with Danica to learn more about how she leveraged an education in physical therapy to work in a cutting-edge field.
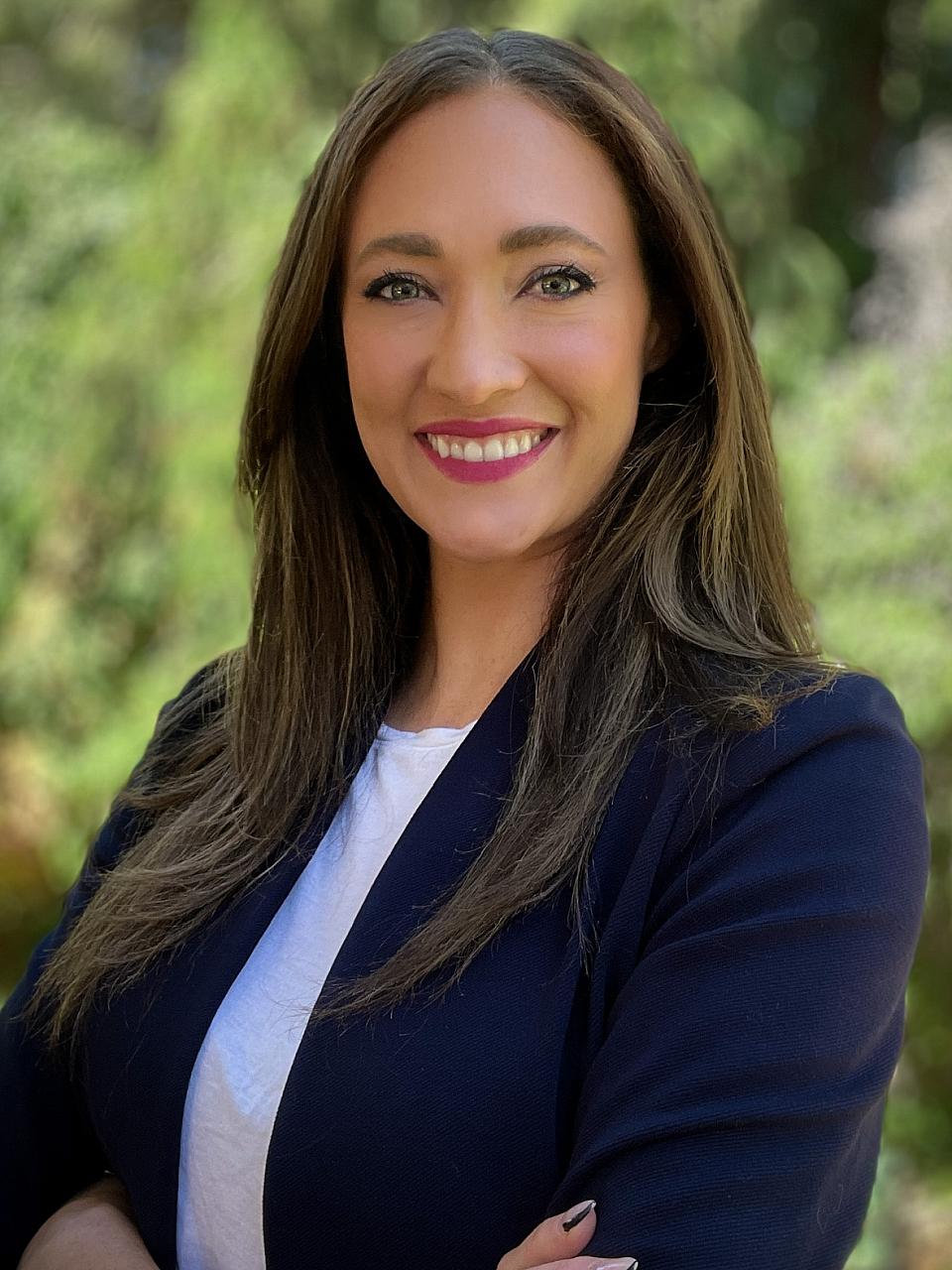
Tell us about your background and how you ended up at the College of Health?
I grew up playing sports and liked being around sports, so I always thought I’d work as a sports PT. I went to Northwestern University for PT school—it is a heavy neuro program and I fell in love with neurorehabilitation. When I was coming to the close of grad school, I knew I wanted to learn more about neuroscience and wanted to do a residency, so I applied all over the country. I applied to Utah because I have family out here and I really like the professors and the vibe of the school.
I did a yearlong neurological physical therapy residency and I got to work in inpatient, outpatient, and rehab. After that I stayed on at U of U Health as a physical therapist for two years. I worked on the Neuro Acute floor and in the Neuro ICU with a large variety of neurological conditions including stroke, traumatic brain injury, spinal cord injury, demyelinating diseases, and brain tumor resections.
I never envisioned going on to do a PhD and leaving clinical care. But when I did my residency and worked in multiple different settings in one organization, I started to get a feel for the inner workings of health care systems. I also noticed some inefficiencies that occurred as patients transfer and it spurred my interest in quality improvement.
Robin Marcus was my mentor for residency, and I joke that she kind of coerced me into doing a PhD. When I completed residency, I met with her about doing a program, but I decided to work in the clinical setting for a while. I kept gravitating to how data can improve trends in care and how we capture information in PT documentation. I reconnected with Robin and entered the PhD program in rehabilitation science. That combined my interests of using healthcare data to inform PT practice.
How did your PhD program prepare you to work in machine learning?
I was able to be part of the translational research and practice program, so I could still work once a week in the clinic while completing my degree. That allowed me to get ideas from my peers about where I could go with my research.
It still took me a while to figure out exactly what I wanted to do with my PhD. About a year and a half into the program, I went to a conference and learned about machine learning and how you could use it to analyze data. I thought it was magic! I found a course in my PhD program that was all about data science, and it led me to my dissertation.
Ultimately, I decided to write my dissertation on predicting post hospital healthcare utilization using machine learning. I wasn’t sure if I wanted to continue to work in a clinic, work as a professor or do something else post-graduation. But I kept gravitating toward impacting clinical care. I came across a position with Medical Group Analytics where I could serve as facilitator between providers and data systems. MGA provides practice-based management and clinical analytics services to our providers and others who staff the practices of the academic faculty.
Tell us about your work now?
Thanks to a huge increase in the popularity of AI tools, I think a lot of providers are super interested in machine learning. But we need to help them along a journey of data literacy, starting at the bottom and working up. Then they know how to use it and what questions it can answer.
For example, we’re working on a project with the Department of Orthopedics. We’re using a patient’s historical data and any patient reported outcome measures to help inform and predict how they’ll do after a procedure. The data can predict their function, and we want to make it usable by providers so they can be even better informed when they meet with patients.
As this field continues to explode, we’re going to get a lot more interest and quality of data will be top of mind. We need to improve how we capture it and capture it more frequently. But ultimately it will expand the prediction space, so we can refine and personalize patients’ health journeys. We want to help patients get back to what they love to do after the optimal number of interventions—and we can use data to predict that.
Are there any downfalls to machine learning?
Data does have bias, and the bias that exists is related to the information we’re not capturing. Salt Lake City and Utah in general have lower minority populations so we don’t have enough data on them. Those are pitfalls that need to be considered and addressed just for representation. Historically minority populations haven’t had equal access to healthcare and that can be reflected in the data. I think the quality of the data we collect is solid, but the main problem are the areas where we can’t collect data.
Where do you see yourself going in this career?
I’ve been with Medical Group Analytics for two years and I’ve been able to work on some awesome projects that are system wide. I do miss clinical care and the impact you make in patients’ lives. But I still feel like I’m able to make an impact on a larger scale. I’d love to help grow our data science program in healthcare. Let’s create more resources for providers and clinicians to help them along their data journey.